Word Vectors
Word embedding is an exciting way to sequence single words into word clusters. Unlike topic models that sort words by usage, “word vectors are about the relationship or closeness of words within a single corpus. Using the word embedding process, it is not necessary to know all the aspects of the algorithm, but “…we do need to understand the mechanics of a transformation.” (Schmidt, 2015)
The allure of web-embedded models is the spatial exploration of word connections. While it was pointed out that Word-Vectors tend not to be used as much as topic models by Digital humanists, the WEM has generated excitement in the machine-learning world. Computers do not define words but can see meaning in the similarities, so they teach the computer about “simile and analogy.” (Schmidt 2015)
On the other hand, WEMs are not very good at dealing with large-scale syntax, but digital humanists can see the strengths in analyzing vocabulary and spatial context. Strangely, computers often show words with the same meaning without knowing the definitions of words by the user of word vectors.
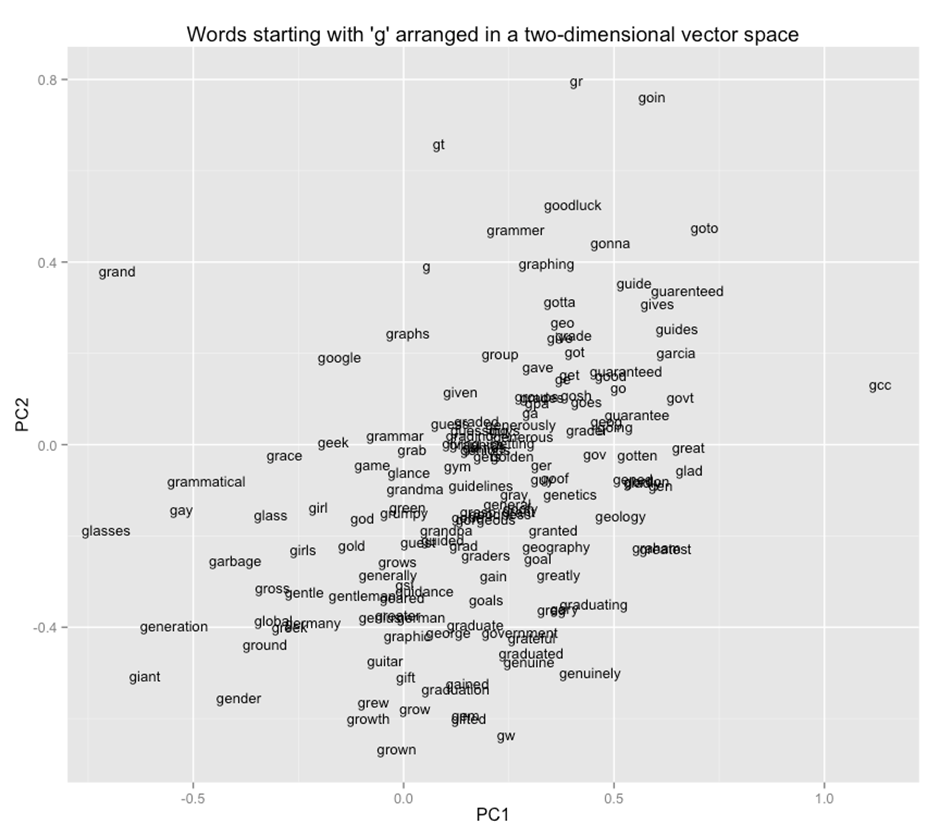
The word vectors on this graph show how closely related words with similar meanings are. Schmidt’s best example was Grandma and Grandma; they are situated closely in the diagram. The vector takes words and organizes them into a 2-dimensional space where they can be studied. Unlike a word cloud, the WEM does not show the most used words but the connections of words. “The goal of a perfect WEM transformation (something that does not exist) is a vector space that can encode all of those relationships simultaneously.” (Schmidt 2015)
Word vectors have the bonus of being easily used over time. As a historian, I find this more valuable, and I admire Sydney Bowens for her creation of “Using Temporal Word Embedding to Reveal the Shifting Notion of Beauty in Vogue.” It is an example of how word embedding models can be used over time. She used a robot’s reading of the magazine published since. December 17, 1892. By analyzing the relationships to “Beauty,” Sydney Bowens could chart what was necessary by decade. I hope to use this in my research; over time, it would be beneficial to analyze the cultural developments within Milltowns. I am studying the aspects of child labor, especially concerning time worked. Children were predominantly used to getting into small places to troubleshoot issues with machines. This was very dangerous. However, web embedding models would greatly help look at policy changes over time.
An example of historians using this application is” Abolitionist Networks: Modeling Language Change in the Nineteenth Century Activist Newspapers,” an article by Sandeep Soni, Lauren F. Klein, and Jacob Eisenstein. They could separate the newspapers and determine semantic changes using diachronic word embedding. As a result, the voices of black women editors became clearer. This also helped to find leaders within the abolition movement. Two specific newspapers, the Provincial Freeman and The Lilly, stood out in the analysis. Black women edited both. These papers led to semantic changes, proving black women were the abolitionist movement’s leaders. This, alongside Syndy Bowen’s web embedding of Vogue magazine, is just two examples of how digital historians could use word vectors.
Ben Schmidt created an application using vector words to show how practical, sometimes amusing, and confusing WEMs can be. In “Gendered Language in Teacher Reviews,” the app is set up to show the connection of male and female teachers to certain words by using data from RateMyProfessor.com. It allows us to rate using all positive or negative ratings. During my class in Digital Historiography, we tinkered with the app and were amazed at the results. For example, a hot professor would be a female engineer, and a crazy professor would most likely be a male art teacher. I thought this might be helpful using gender, age, and assigned jobs from Milltown. I want to take the transcribed interviews of Mill workers in the University of North Carolina at Chapel Hill’s archives. At the same time, they have thousands of interviews, which would be a corpus of about three hundred oral history interviews. I could start the process by distinguishing similar negative and positive words in a data set. This would be a cornerstone of the research. Analyzing both positive and negative will give some idea of the attitudes of the mill workers. I was not as excited about topic model results. While they were easier to interpret by a glance, having an application that can aid in analyzing over time and show actual negative and positive outlooks will fit more into the study of digital history, in my view.
This article was a great read! It managed to break down complex ideas into easily understandable concepts. Im really interested in seeing how this topic evolves. For those who want to delve deeper, check out my profile by clicking on my nickname!
This is the fitting weblog for anybody who wants to search out out about this topic. You realize so much its virtually exhausting to argue with you (not that I truly would need…HaHa). You undoubtedly put a brand new spin on a subject thats been written about for years. Great stuff, just great!